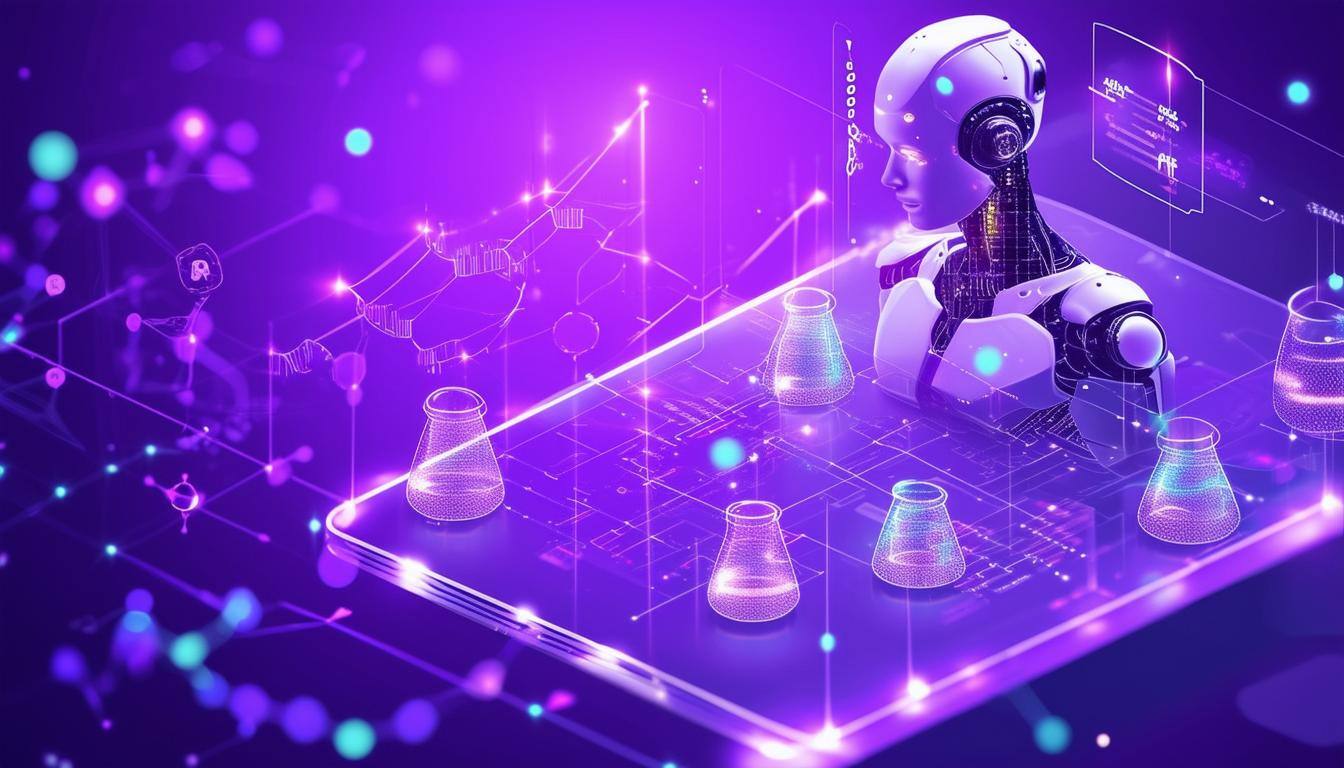
The single tactic every clinical development & Integrated evidence plan should have

A Not So Tired Cliche:
Drug development is a discipline where we are often asked to expect the unexpected, between a potential unexpected safety event appearing in an early phase clinical study through to significantly slower than projected patient recruitment, development teams have to navigate and manage uncertainty while under significant time pressures.
The tired adage that is quoted ad nauseam by those often with MBA in their title of "being proactive as opposed to reactive" can only apply so far in drug development given our need to generate data to inform our decisions.
That said, there is a single tactic that if used correctly both fundamentally changes how we respond to uncertainty and also significantly reduce the time it takes to make the best decision for a development program.
Introducing The Data Landscaping Study:
While this sounds like an anticlimax, I can assure you that a data landscaping study is often the missing component that causes untold delays and issues in many clinical development plans and integrated evidence plans.Put simply, a data landscaping study is essentially a global, systematic review of all relevant real-world data sources for a specific disease that answers a very simple set of questions:
- Where is the data relevant to my study located?
- What elements does the data contain (OS, PFS, ORR, lab values etc)?
- Who is the custodian of this data?
While sounding deceptively simple, data landscaping studies involve a comprehensive and meticulous examination of a vast array of data sources. This process requires a deep understanding of the nuances and complexities inherent in real-world data, as well as the ability to navigate the challenges of data integration and interpretation.
But the question remains: Why should every clinical development plan and integrated evidence plan include a data landscaping study from day one?
Rapid Response: Data Landscaping Guiding Clinical Development
Many years ago I worked on an early phase rare disease program that was considered to be integral to the company's pipeline strategy. During the conduct of the trial we had to establish whether a group of adverse events were indeed due to the study drug or a function of the underlying disease.
While this is not an uncommon occurrence in the life of a drug developer, what made this situation unique was that there was no scientific consensus within the medical community on what the baseline risk for the given event was in this rare disease population.
Thanks to a proactive data landscaping and data acquisition strategy during early clinical development, the team were perfectly positioned to address this question thoroughly and in a relatively short period of time.
Using data of thousands of patients who matched the population in the trial, the team were able to model and understand what the underlying rate of the event of interest was and adjust development accordingly.
Without a data landscaping study already having been conducted, the above situation would have taken significantly longer to address.
Data Landscaping Studies Turbo Charge Trial Design
During early clinical development we're often juggling several questions: which endpoint should we consider, what comparator should we use, what should the treatment effect estimate be?
While we often lean on our (and the teams') experience to set a frame to address several aspects of study design, the end result remains the same: we need to generate assumptions that may or many not be bourn out in reality.
Relatively early in my career, I was on a team developing a randomised trial for an oncology asset. The team were as certain as could be on the standard of care (SOC) definition and had already pressure tested it with some opinion leaders. However, thanks to a proactive data landscape and acquisition strategy we were able to verify the SOC was using RWD within a week.
The RWD analysis showed that had we kept our original SOC definition, the trial would have run into severe recruitment challenges and as a result, the SOC arm was updated.
The above example provides the key value proposition for a data landscaping study: it's a tool that fundamentally accelerates clarity in drug development.
- Highlighting the potential issues in trial inclusion criteria on recruitment.
- Highlighting potential issues with a treatment effect assumption that could have resulted in a missed endpoint.
- Showing how the natural history of a biomarker derived cohort deviated from assumptions about the general condition.
Data Landscaping Studies Accelerate Innovation:
The best development teams I've had the fortune of being part of have had one thing in common: The team were always a tinderbox of ideas. Irrespective of the challenge that emerged, we were able to rally together as a team and through a sprint, propose a pragmatic solution.
A data landscaping study fosters innovation in drug development by making teams aware of all available global data that can address a given question. By having data identified and meta-data extracted, questions shift from "If the data existed we could do this" to "given the data, here are our options".
This shift means ideation becomes both pragmatic and efficient - by having all relevant data tagged and identified, teams' are more likely to engage with it and make truly data driven decisions.
As another practical example, during an early-phase program, our development team and I concluded that a single-arm trial with an external control arm (ECA) was likely the only feasible way to demonstrate our asset’s comparative efficacy. While the data landscaping study quickly helped us identify a suitable source for our ECA, its advantages went well beyond that. During regulatory interactions, the landscaping study not only allowed us to justify the scientific rationale for selecting an ECA, but also provided supplementary data supporting our position that a randomised study would not be feasible.
Conclusion
Data landscaping studies are invaluable strategic tools that empower drug development teams to address questions as they arise. They reduce the uncertainties that drug developers have to contend with and significantly enhance decision making.