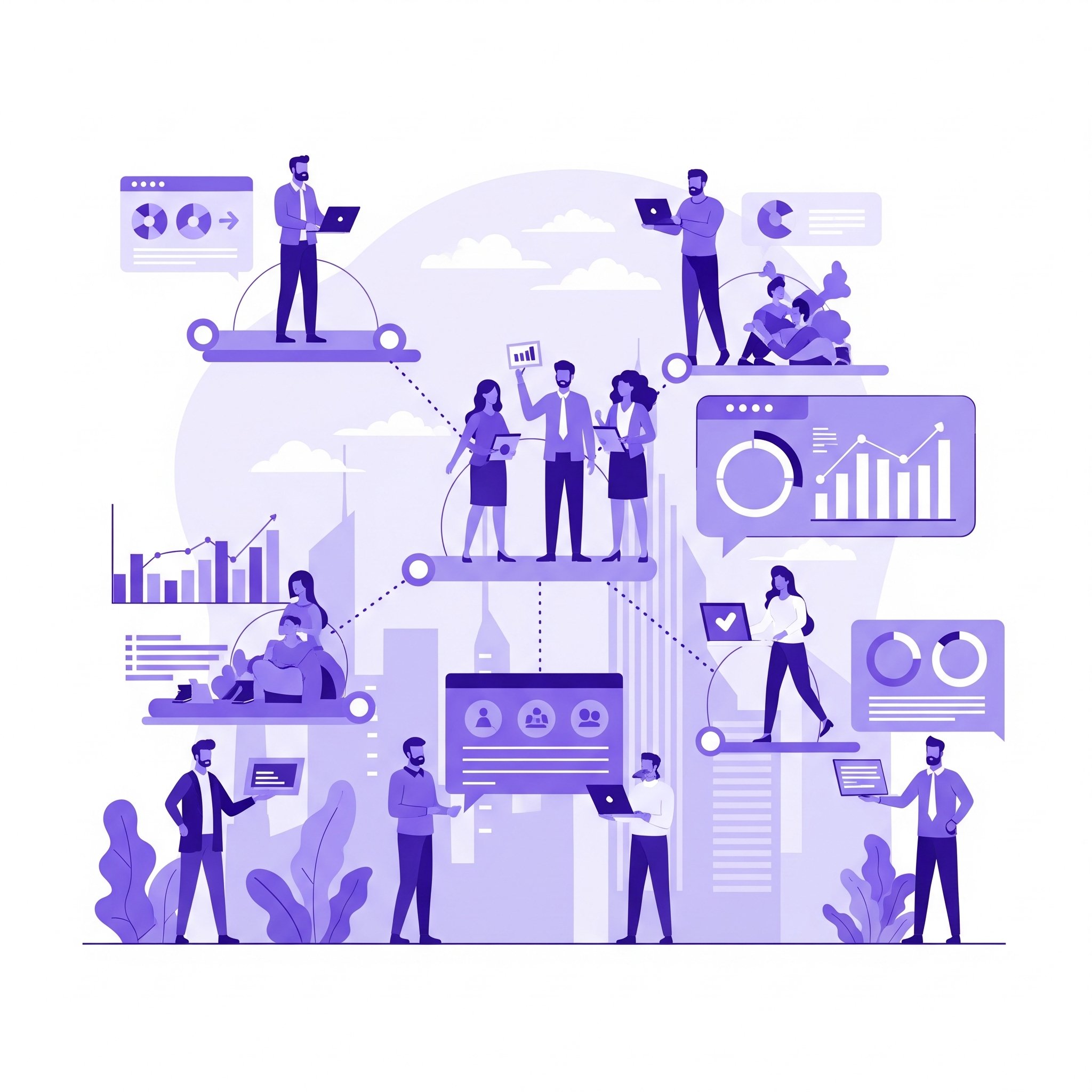
How will FDA’s new AI draft guidance impact Drug Development?
)%20(1).png?width=64&height=64&name=Untitled%20(Book%20Creator%20Cover%20(Square))%20(1).png)
The FDA’s draft guidance on the use of artificial intelligence (AI) in regulatory decision-making for drugs and biologics represents a key step in defining how AI can be integrated into the drug development and regulatory landscape. The document lays out a structured, risk-based credibility assessment framework that companies must follow when submitting AI-generated evidence to the FDA. This approach is designed to ensure that AI models used in regulatory submissions are trustworthy, transparent, and appropriately validated.
The FDA’s AI Credibility Framework: A Seven-Step Process
The guidance provides a seven-step risk-based framework to assess the credibility of AI models used to support regulatory decision-making. These steps serve as a structured process for AI developers and pharmaceutical companies to validate their AI models.
1. Defining the Question of Interest
- The AI model's role and purpose must be clearly articulated, particularly in the context of drug development and regulatory decision-making.
- AI should be used to answer specific, well-defined regulatory questions (e.g., “Can AI help stratify patient risk in a clinical trial?”).
2. Defining the Context of Use (COU)
- The specific role and scope of an AI model within the regulatory process must be established.
- Companies must specify whether the AI model is used alone or alongside human judgment or other data sources in decision-making.
- Example: If an AI model is used to predict patient safety risks in a trial, the COU should define the data sources, model function, and how its output is integrated into the clinical workflow.
3. Assessing AI Model Risk
- The FDA proposes a risk matrix based on two primary factors:
- Model Influence – How heavily the AI model’s outputs are weighted in regulatory decision-making.
- Decision Consequence – The potential impact of an incorrect AI decision on patient safety, drug efficacy, or product quality.
- High-risk models (e.g., AI models making direct safety assessments for trial participants) will require more rigorous validation than lower-risk applications (e.g., AI models optimizing administrative processes in drug development).
4. Developing a Credibility Assessment Plan
- AI developers must create a formal validation plan detailing:
- How the AI model was built
- What data was used
- How biases were minimized
- How model performance will be evaluated over time
- The plan should be tailored to the AI model’s risk level, with higher-risk models requiring more stringent validation and oversight.
5. Executing the Plan
- Companies must implement the validation plan as part of their AI deployment.
- AI models should be rigorously tested and refined based on the predefined credibility assessment strategy.
6. Documenting Outcomes & Deviations
- AI models must maintain detailed records of their performance, including:
- Training data sources
- Bias mitigation strategies
- Any deviations from the original validation plan
- Transparency is a key requirement; companies must demonstrate that their AI models remain reliable over time.
7. Determining AI Model Adequacy
- AI developers must periodically reassess whether the model remains fit for purpose.
- Any changes in data inputs or clinical context must trigger a re-evaluation of AI credibility.
Key Challenges & Considerations Highlighted in the Guidance
While AI holds enormous potential for accelerating drug development, the FDA identifies several risks that companies must address:
Data Quality & Bias Concerns
- AI models rely heavily on training data, but biases in datasets (e.g., underrepresentation of certain patient groups) can lead to flawed conclusions.
- The FDA emphasizes that AI models must be trained on representative, high-quality data that aligns with the regulatory "fit for use" standard.
Model Explainability & Transparency
- The lack of interpretability in some AI models, particularly deep learning models, presents regulatory challenges.
- The FDA expects companies to clearly document how AI models reach conclusions and provide mechanisms for human oversight.
Lifecycle Maintenance & AI Model Drift
- AI models must be continuously monitored to ensure that they remain accurate and do not drift when exposed to new data.
- The FDA requires companies to implement ongoing model validation processes to detect performance degradation.
Early FDA Engagement: A Must for AI Success
The FDA is actively encouraging sponsors to consult early when using AI, offering engagement opportunities through:
Complex Innovative Trial Design (CID) Program – For integrating AI into novel trial designs.
Emerging Drug Safety Technology Program (EDSTP) – Focused on AI in post-marketing safety monitoring.
Real-World Evidence (RWE) Program – Evaluating AI applications in real-world data analysis.
Early alignment with the FDA is critical to ensuring AI models meet regulatory expectations and avoid late-stage rejection.
What will this mean for Drug Developers & AI Vendors
Although the guidance is currently in draft form, it indicates an evolving, mature regulatory approach to AI, where high-risk AI models will require formal validation and transparency before being accepted in regulatory decision-making. Companies using AI in drug development should take the following steps:
-
Establish Robust AI Governance:
- Implement internal frameworks for AI validation, documentation, and continuous oversight.
- Adopt best practices for AI model risk assessment and bias mitigation.
-
Align AI Model Use with Regulatory Expectations:
- AI applications in clinical trials, safety assessments, and manufacturing will face higher regulatory scrutiny.
- Companies should tailor AI deployment strategies to align with FDA credibility standards.
-
Engage Regulators Early:
- AI model developers should proactively consult the FDA before using AI for regulatory submissions.
- Companies should leverage the FDA’s risk framework to demonstrate compliance and build trust in AI-driven decisions.
Conclusion: A New Era of AI Oversight in Pharma
The FDA’s draft guidance sets a precedent for AI adoption in drug development, balancing innovation with patient safety and product integrity. Rather than restricting AI’s role, the agency is providing a structured approach to ensure AI models used in regulatory decision-making are credible, transparent, and fit for purpose.
Especially for AI vendors, this is a wake-up call—AI must go beyond the hype curve and be accurate and credible. Pharma and Biotechnology organizations that prioritize robust validation, proactive regulatory engagement, and ongoing monitoring will be best optimally positioned to integrate AI effectively into drug development and regulatory processes.
This guidance also signals that AI will continue to play a critical role in advancing drug development—but with a growing emphasis on governance, lifecycle management, and human oversight. Companies that align their AI strategies with the FDA’s risk-based framework will not only accelerate regulatory acceptance but also enhance the trust and reliability of AI-driven insights.